Juhtimissüsteemide andmeteaduse uurimisrühm
Data Science for control systems research group
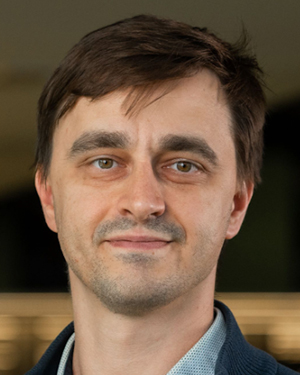
Tallinn University of Technology,
School of Information Technologies,
Department of Software Science
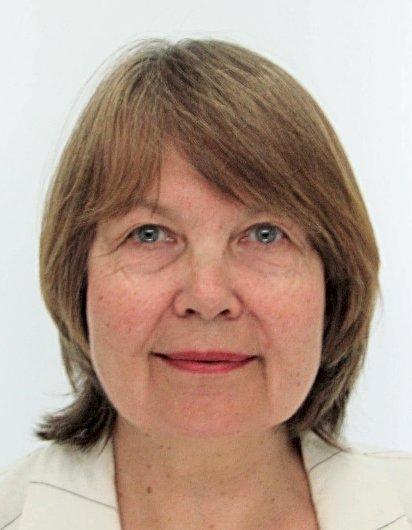
Lead. researcher Ülle Kotta
Tallinn University of Technology,
School of Information Technologies,
Department of Software Science
Introduction
This research group is a part of a larger Nonlinear Control Systems group and is focused on developing advanced IT solutions to support digital transformation of the energy domain. Professor Juri Belikov is responsible for teaching of data science methods in energy domain. With 5 active PhD students and some senior researchers, the total number of personnel is about 10 persons. With other groups, there is intensive cooperation with prof. Eduard Petlenkov regarding intelligent and data-driven control systems and lead. researcher Dmitri Vinnikov regarding energy efficient and smart electrification technologies.
Competence
Data Science research group represents key competencies and teaching responsibilities in the following domains:
• Data-driven modelling and analysis
• Trustworthy (eXplainable) AI and machine learning methods
• Energy efficient and optimal solutions
• Mathematical control theory of nonlinear systems
While our primary focus has been on developing application-independent general methods driven by the dynamic properties of mathematical models, we have recently directed our attention towards a few select applications. In particular, we put an emphasis on addressing both theoretical and practical challenges associated with digital transformation of energy domain. This work has been conducted through the lens of advanced IT technologies such as Artificial Intelligence and Machine learning, leading to the emergence of a unique research field in Estonia known as ‘Energy Informatics’. This interdisciplinary domain combines our expertise in control systems with cutting-edge information technology, facilitating innovative solutions for energy management and sustainability.
Moreover, data science has become an indispensable tool in our research, particularly for energy applications within smart buildings. By leveraging advanced data analytics, machine learning, and big data technologies, we developed novel solutions to optimize energy consumption and management in smart buildings. These data-driven approaches enable us to monitor and control building systems more effectively, predict energy usage patterns, and enhance the overall energy efficiency of buildings. The integration of data science and control systems in smart buildings opens new avenues for innovation, contributing significantly towards creating more sustainable and intelligent living and working environments.
Some examples of research topics and outcomes:
• Differential algebraic framework of one-forms for the analysis of structural properties of nonlinear control systems;
• Explainable AI tools for PQD, FDD, and NILM problems;
• Novel control algorithms for optimal control of energy storage systems under limited information and uncertainty conditions;
• Modelling and analysis of large scale networks based on dq0 frame of reference;
• Innovative energy services based on behavioural – reflective – attributes and intelligent recommendation systems.